Researchers find that promises of market-beating performance and distinctive portfolios go unfulfilled
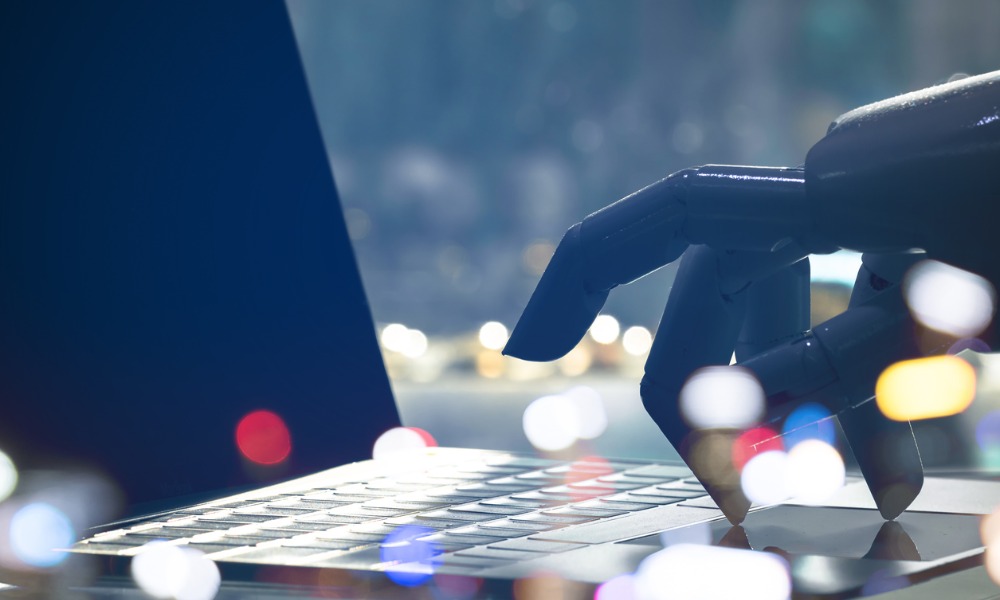
We’re two decades into the twenty-first century, and over that time technology has continued to be a disruptive force across industries. But when it comes to the use of artificial intelligence (AI) in stock-picking, it seems the gap between potential and real market-beating returns has yet to be bridged.
Citing research done by Doron Avramov, a finance professor at the Interdisciplinary Center Herzliyah in Israel, the Wall Street Journal reported that AI strategies that were tested using historical data generated phenomenal paper returns, besting the market by as much as 40% on an annualized basis.
Those kinds of returns are certainly seductive when one considers the emerging picture in factor-based investing. “Researchers have found that more than half of the paper profit that initial studies reported for [factors such as momentum, market cap, volatility, low ratios of price to earnings, book value, and sales] disappeared when they were put into practice,” the Journal said.
That begs the question: are the returns that emerged from tests of AI strategies any more robust than those for factors? That’s addressed by Avramov, along with two colleagues from the Chinese University of Hong Kong and the Hebrew University of Jerusalem, in a recently completed study.
The group recreated several different neural networks and other machine-learning techniques that past AI researchers have found to be promising. After incorporating virtually all indicators that previous studies found to be of some value in stock-picking — more than 100 in total — they used a database of U.S. stocks going back to 1957 to “train” the networks. Different interactions and combinations of the indicators were tested in an attempt to generate performance that went beyond what the indicators could produce individually.
“[T]hey noticed that much of the portfolios’ paper profits were coming from microcaps—stocks with tiny market caps,” the Journal report said, noting that managers can’t establish sizeable positions in such stocks without significantly pushing up their prices. “It’s also difficult to borrow shares of these stocks when you want to sell them short.”
Avramov and his colleagues then restricted the AI strategies to more liquid stocks that were relatively easy and cheap to trade. With that tweak, they found that more than half of the strategies’ paper profits evaporated; transaction costs were also cited as a concern, given that approaches built on machine learning tend to generate much higher trading volumes than conventional factor-based strategies.
Another problem, reported the Journal, is that the portfolios yielded by the AI strategies weren’t particularly special. Citing Avramov, it said that they were actually quite similar to portfolios produced by well-known factors.
“This perhaps helps to explain why hedge funds that employ AI haven’t outperformed the S&P 500 over the last decade,” the Journal said, noting that the Eurekahedge AI Hedge Fund Index has produced a 12.7% annualized return since its January 2010 inception, compared to the S&P 500’s 13.3% dividend-adjusted return.