Outcome Wealth Management takes on underperforming managers with AI, machine learning strategies
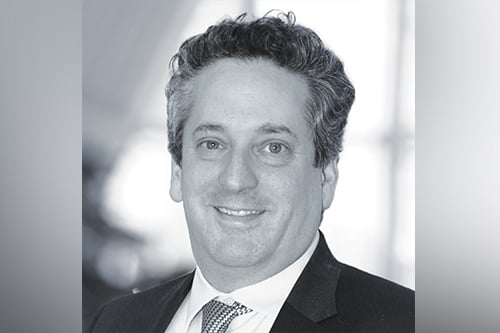
When it comes to big data analysis, Noah Solomon is reminiscent of those classic toy cars; just wind him up and watch him go. It’s a comparison that could also be applied to his company Outcome Wealth Management, which is starting to make its mark among investors.
The president and CIO is a passionate believer in his AI, machine-learning approach to investing, with Outcome reaching $50 million AUM in two years through direct clients, high-net-worth individuals and family offices. It’s poised to open its funds up to advisors and is in talks with various banks to add to its current availability with Royal Bank.
Solomon is also not afraid to ruffle feathers to get his point across and beleives portfolio manager’s over-reliance on human interaction and subjective interpretation is flawed.
By taking on underperforming active managers with its two funds – a core strategy based on liquid index-tracking ETFs and a Canadian dividend focused equity strategy – Outcome aims to maximize big data analysis and take human emotion out of the equation.
Driving this forward, Solomon brings with him vast experience in the investment world. From 2008 to 2016, he was CEO and CIO of GenFund Management (formerly Genuity Fund Management), where he designed and managed data-driven, statistically based equity funds, and before that he was a proprietary trader in the equities division of Goldman Sachs.
Solomon explained the genesis of Outcome to WP through two prisms – performance and how you get there.
The former is about “harnessing the magic of compounding”, which means avoiding large losses. Losing 50%, for example, requires you to make 100% to get back to where you started. The strategy, therefore, aims to participate in a rising market – "not necessarily knock the lights out" – but more importantly protect investors every five to 10 years in a bear market.
This involves hedge fund characteristics but without the baggage this usually entails, like high management fees and relatively poor liquidity (monthly or quarterly notice periods). Solomon added: “If a client wants their money out for whatever reason, to buy a cottage or move to Bhutan, whatever it is, we have weekly liquidity, one day's notice.”
Then it’s a case of how you get there – and that’s when big data analysis and AI really enters the picture. Outcome’s view of behavioural economics is that the human attributes that have enabled us to thrive as a species are terrible for investment decision-making, and that this is true for Joe Blow in the East End to professional institutional money managers on Bay Street, who are likely to lean towards stocks that have worked for them before.
Solomon said: “We all know from the data that at least 90% of active managers underperform their benchmarks over the long term; that is a fact, we have the data.
“I like AI for what it doesn't have. It doesn't have emotions; it can't read someone's body language, but so what? I also like it for what it does have, what it can do that a human being can’t in the volume of data it can analyze and its pattern recognition capabilities. Only a powerful computer and algorithms can do that.”
Flat during December downturn
Of course, the proof is in the pudding but Solomon told WP both strategies are outperforming. The core strategy is tactical with its ETF mix – it can be all equity, all bonds or anywhere in between. It’s entirely algorithmically based and the CIO said it has produced a risk-adjusted return very few have matched since its inception two and a half years ago. He proudly pointed out that it was flat in the last quarter of last year.
Solomon said: “Our artificial intelligence or machine-derived learning based signals started to pick up some noise at the end of September, and liquidated all its equity exposure. We were 100% in short-term investment grade corporate bond ETFs, basically cash, so we avoided losses.
“The funny thing is, you take a year like this where equity markets are doing pretty well, and people are so happy, saying ‘I'm making money’. They're not making money, they’re just getting their money back, whereas we're actually making money because we started with 100 cent dollars, not 80 cent dollars.”
The firm’s second strategy is also based on machine-learning derived signals and formulas and is a Canadian dividend focused equity strategy. It’s 100% long all of the time and was borne out of clients wanting an income or yield via Canadian dividends, which have a tax break.
Outcome looked at the three largest Canadian dividend funds in the country and found that, for the most part, they were bank-managed mandates with billions of dollars across them. When compared to the top-10 weightings in the TSX, the top 10 in those three funds had six in common. Solomon, therefore, was armed with the evidence that they were all doing the same thing and were, in effect, closet indexers. Further reseach, via SPIVA, revealed that managers in the space had managed to outperform the TSX Dividend Aristocrats index 0% of the time.
“That was curious to us because you have this area that is in huge demand, because people want income and they'd like it tax preferred. Yet, it seemed to be an area where managers struggle to differentiate themselves or add value.
“We were either stupid enough, crazy enough or bold enough to apply our algorithms to this problem. We went to the data room, submerged ourselves for about four months and came up with a long Canadian dividend focused strategy. It’s only been running for about a year, but it has gotten both higher returns, and lower volatility, than the TSX Dividend Aristocrats or the TSX.”